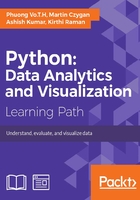
Computational tools
Let's start with correlation and covariance computation between two data objects. Both the Series and DataFrame have a cov
method. On a DataFrame object, this method will compute the covariance between the Series inside the object:
>>> s1 = pd.Series(np.random.rand(3)) >>> s1 0 0.460324 1 0.993279 2 0.032957 dtype: float64 >>> s2 = pd.Series(np.random.rand(3)) >>> s2 0 0.777509 1 0.573716 2 0.664212 dtype: float64 >>> s1.cov(s2) -0.024516360159045424 >>> df8 = pd.DataFrame(np.random.rand(12).reshape(4,3), columns=['a','b','c']) >>> df8 a b c 0 0.200049 0.070034 0.978615 1 0.293063 0.609812 0.788773 2 0.853431 0.243656 0.978057 0.985584 0.500765 0.481180 >>> df8.cov() a b c a 0.155307 0.021273 -0.048449 b 0.021273 0.059925 -0.040029 c -0.048449 -0.040029 0.055067
Usage of the correlation method is similar to the covariance method. It computes the correlation between Series inside a data object in case the data object is a DataFrame. However, we need to specify which method will be used to compute the correlations. The available methods are pearson
, kendall
, and spearman
. By default, the function applies the spearman
method:
>>> df8.corr(method = 'spearman') a b c a 1.0 0.4 -0.8 b 0.4 1.0 -0.8 c -0.8 -0.8 1.0
We also have the corrwith
function that supports calculating correlations between Series that have the same label contained in different DataFrame objects:
>>> df9 = pd.DataFrame(np.arange(8).reshape(4,2), columns=['a', 'b']) >>> df9 a b 0 0 1 1 2 3 2 4 5 3 6 7 >>> df8.corrwith(df9) a 0.955567 b 0.488370 c NaN dtype: float64