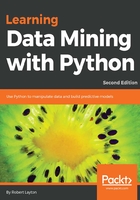
Affinity analysis
Affinity analysis is the task of determining when objects are used in similar ways. In the previous chapter, we focused on whether the objects themselves are similar - in our case whether the games were similar in nature. The data for affinity analysis is often described in the form of a transaction. Intuitively, this comes from a transaction at a store—determining when objects are purchased together as a way to recommend products to users that they might purchase.
However, affinity analysis can be applied to many processes that do not use transactions in this sense:
- Fraud detection
- Customer segmentation
- Software optimization
- Product recommendations
Affinity analysis is usually much more exploratory than classification. At the very least, we often simply rank the results and choose the top five recommendations (or some other number), rather than expect the algorithm to give us a specific answer.
Furthermore, we often don't have the complete dataset we expect for many classification tasks. For instance, in movie recommendation, we have reviews from different people on different movies. However, it is highly unlikely we have each reviewer review all of the movies in our dataset. This leaves an important and difficult question in affinity analysis. If a reviewer hasn't reviewed a movie, is that an indication that they aren't interested in the movie (and therefore wouldn't recommend it) or simply that they haven't reviewed it yet?
Thinking about gaps in your datasets can lead to questions like this. In turn, that can lead to answers that may help improve the efficacy of your approach. As a budding data miner, knowing where your models and methodologies need improvement is key to creating great results.