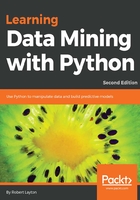
Summary
In this chapter, we extended our use of scikit-learn's classifiers to perform classification and introduced the pandaslibrary to manage our data. We analyzed real-world data on basketball results from the NBA, saw some of the problems that even well-curated data introduces, and created new features for our analysis.
We saw the effect that good features have on performance and used an ensemble algorithm, random forests, to further improve the accuracy. To take these concepts further, try to create your own features and test them out. Which features perform better? If you have trouble coming up with features, think about what other datasets can be included. For example, if key players are injured, this might affect the results of a specific match and cause a better team to lose.
In the next chapter, we will extend the affinity analysis that we performed in the first chapter to create a program to find similar books. We will see how to use algorithms for ranking and also use an approximation to improve the scalability of data mining.