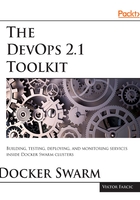
Problems when scaling stateful instances
Scaling services inside a Swarm cluster is easy, isn't it? Just execute docker service scale <SERVICE_NAME>=<NUMBER_OF_INSTANCES> and, all of a sudden, the service is running multiple copies.
The previous statement is only partly true. The more precise wording would be that "scaling stateless services inside a Swarm cluster is easy".
The reason that scaling stateless services is easy lies in the fact that there is no state to think about. An instance is the same no matter how long it runs. There is no difference between a new instance and one that run for a week. Since the state does not change over time, we can create new copies at any given moment, and they will all be exactly the same.
However, the world is not stateless. State is an unavoidable part of our industry. As soon as the first piece of information is created, it needs to be stored somewhere. The place we store data must be stateful. It has a state that changes over time. If we want to scale such a stateful service, there are at least two things we need to consider:
- How do we propagate a change of state of one instance to the rest of the instances?
- How do we create a copy (a new instance) of a stateful service, and make sure that the state is copied as well?
We usually combine stateless and stateful services into one logical entity. A back-end service could be stateless and rely on a database service as an external data storage. That way, there is a clear separation of concerns and a different lifecycle of each of those services.
Before we proceed, I must state that there is no silver bullet that makes stateful services scalable and fault-tolerant. Throughout the book, I will go through a couple of examples that might, or might not, apply to your use case. An obvious, and very typical example of a stateful service is a database. While there are some common patterns, almost every database provides a different mechanism for data replication. That, in itself, is enough to prevent us from having a definitive answer that would apply to all. We'll explore scalability of a MongoDB later on in the book. We'll also see an example with Jenkins that uses a file system for its state.
The first case we'll tackle will be of a different type. We'll discuss scalability of a service that has its state stored in a configuration file. To make things more complicated, the configuration is dynamic. It changes over time, throughout the lifetime of the service. We'll explore ways to make HAProxy scalable.
If we use the official HAProxy (https://hub.docker.com/_/haproxy/) image, one of the challenges we would face is deciding how to update the state of all the instances. We'd have to change the configuration and reload each copy of the proxy.
We can, for example, mount an NFS volume on each node in the cluster and make sure that the same host volume is mounted inside all HAProxy containers. At first, it might seem that that would solve the problem with the state since all instances would share the same configuration file. Any change to the config on the host would be available inside all the instances we would have. However, that, in itself, would not change the state of the service.
HAProxy loads the configuration file during initialization, and it is oblivious to any changes we might make to the configuration afterward. For the change of the state of the file to be reflected in the state of the service, we'd need to reload it. The problem is that instances can run on any of the nodes inside the cluster. On top of that, if we adopt dynamic scaling (more on that later on), we might not even know how many instances are running. So we'd need to discover how many instances we have, find out on which nodes they are running, get IDs of each of the containers, and, only then, send a signal to reload the proxy. While all this can be scripted, it is far from an optimum solution. Moreover, mounting an NFS volume is a single point of failure. If the server that hosts the volume fails, data is lost. Sure, we can create backups, but they would only provide a way to restore lost data partially. That is, we can restore a backup, but the data generated between the moment the last backup was created, and the node failure would be lost.
An alternative would be to embed the configuration into HAProxy images. We could create a new Dockerfile that would be based on haproxy and add the COPY instruction that would add the configuration. That would mean that every time we want to reconfigure the proxy, we'd need to change the config, build a new set of images (a new release), and update the proxy service currently running inside the cluster. As you can imagine, this is also not practical. It's too big of a process for a simple proxy reconfiguration.
Docker Flow Proxy uses a different, less conventional, approach to the problem. It stores a replica of its state in Consul. It also uses an undocumented Swarm networking feature (at least at the time of this writing).