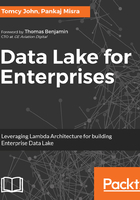
Enterprise-level log analysis
One of the very common use cases for this pattern is log ingestion and various analytics that surround it. The ELK (Elasticsearch, Logstash, Kibana) stack is a leading one in this space, but this pattern could very well be used. The logs can vary from conventional application logs to different types of logs produced by various software and hardware components. If we need to have an enterprise level log management and analytical capability this pattern is indeed a good choice. These logs are produced in large quantities and at very high velocity. Also these are immutable in nature and does need to have an order in place for analyst (may be a developer of an application or a security data scientists making use of this data to decipher any security threat) to make use of these.
Using the important layer in Lambda Layer (batch and speed layer which we will explain in detail in next coming sections) helps validate these real-time logs and also can comprehend past logs to give a real-time insight. These insights can result in some actions which can be proactively assigned to a development team. For instance, application bugs can be assigned to application developer and threat reviews can be assigned to security analysts.
In addition to log analysis to detect certain anomalies, we can also use this data as a analysis methodology and auditing. For example website like, Google Analytics data when fed to lake and analyzed can be used to derive some trends, which can be advantageous to the business running the website.