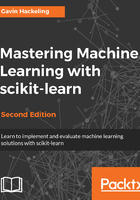
Lazy learning and non-parametric models
KNN is a lazy learner. Also known as instance-based learners, lazy learners simply store the training dataset with little or no processing. In contrast to eager learners such as simple linear regression, KNN does not estimate the parameters of a model that generalizes the training data during a training phase. Lazy learning has advantages and disadvantages. Training an eager learner is often computationally costly, but prediction with the resulting model is often inexpensive. For simple linear regression, prediction consists only of multiplying the learned coefficient by the feature, and adding the learned intercept parameter. A lazy learner can predict almost immediately, but making predictions can be costly. In the simplest implementation of KNN, prediction requires calculating the distances between a test instance and all training instances.
In contrast to most of the other models that we will discuss, KNN is a non-parametric model. A parametric model uses a fixed number of parameters, or coefficients, to define the model that summarizes the data. The number of parameters is independent of the number of training instances. Non-parametric may seem to be a misnomer, as it does not mean that the model has no parameters; rather, non-parametric means that the number of parameters of the model is not fixed, and may grow with the number of training instances.
Non-parametric models can be useful when training data is abundant and you have little prior knowledge about the relationship between the response and explanatory variables. KNN makes only one assumption: instances that are near each other are likely to have similar values of the response variable. The flexibility provided by non-parametric models is not always desirable; a model that makes assumptions about the relationship can be useful if training data is scarce or if you already know about the relationship.