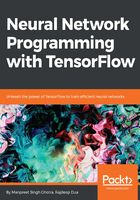
Defining feedforward networks
Deep feedforward networks, also called feedforward neural networks, are sometimes also referred to as Multilayer Perceptrons (MLPs). The goal of a feedforward network is to approximate the function of f∗. For example, for a classifier, y=f∗(x) maps an input x to a label y. A feedforward network defines a mapping from input to label y=f(x;θ). It learns the value of the parameter θ that results in the best function approximation.
We discuss RNNs in Chapter 5, Recurrent Neural Networks. Feedforward networks are a conceptual stepping stone on the path to recurrent networks, which power many natural language applications. Feedforward neural networks are called networks because they compose together many different functions which represent them. These functions are composed in a directed acyclic graph.
The model is associated with a directed acyclic graph describing how the functions are composed together. For example, there are three functions f(1), f(2), and f(3) connected to form f(x) =f(3)(f(2)(f(1)(x))). These chain structures are the most commonly used structures of neural networks. In this case, f(1) is called the first layer of the network, f(2) is called the second layer, and so on. The overall length of the chain gives the depth of the model. It is from this terminology that the name deep learning arises. The final layer of a feedforward network is called the output layer.

These networks are called neural because they are inspired by neuroscience. Each hidden layer is a vector. The dimensionality of these hidden layers determines the width of the model.