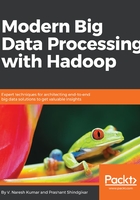
Shuffling
This is also considered one of the standard techniques of achieving anonymity of data. This process is more applicable where we have records of data with several attributes (columns in database terminology). In this technique, the data in the records is shuffled around a column so as to make sure that the record-level information is changed. But statistically, the data value remains the same in that column.
Example: When doing an analysis on the salary ranges of an organization, we can actually do a shuffle of the entire salary column, where the salaries of all the employees never match reality. But we can use this data to do an analysis on the ranges.
Complex methods can also be employed in this case, where we can do a shuffle based on other fields such as seniority, geography, and so on. The ultimate objective of this technique is to preserve the meaning of the data and, at the same time, make it impossible to discover the original owners of these attributes.
Let's see this with some example data:

There are five sample employee records with their salary information. The top table has original salary details and the table below has shuffled salary records. Look at the data carefully and you will understand. Remember that while shuffling, a random algorithm can be applied to increase the complexity of discovering the truth.