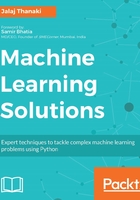
Understanding the datasets
Finding out an appropriate dataset is a challenging task in data science. Sometimes, you find a dataset but it is not in the appropriate format. Our problem statement will decide what type of dataset and data format we need. These kinds of activities are a part of data wrangling.
Note
Data wrangling is defined as the process of transforming and mapping data from one data form into another. With transformation and mapping, our intention should be to create an appropriate and valuable dataset that can be useful in order to develop analytics products. Data wrangling is also referred to as data munging and is a crucial part of any data science application.
Generally, e-commerce datasets are proprietary datasets, and it's rare that you get transactions of real users. Fortunately, The UCI Machine Learning Repository hosts a dataset named Online Retail. This dataset contains actual transactions from UK retailers.
Description of the dataset
This Online Retail dataset contains the actual transactions between December 1, 2010 and December 9, 2011. All the transactions are taken from the registered non-store online retail platform. These online retail platforms are mostly based in the UK. The online retail platforms are selling unique all-occasion gifts. Many consumers of these online retail platforms are wholesalers. There are 532610 records in this dataset.
Attributes of the dataset
These are the attributes in this dataset. We will take a look at a short description for each of them:
- InvoiceNo: This data attribute indicates the invoice numbers. It is a six-digit integer number. The records are uniquely assigned for each transaction. If the invoice number starts with the letter 'c', then it indicates a cancellation.
- StockCode: This data attribute indicates the product (item) code. It is a five-digit integer number. All the item codes are uniquely assigned to each distinct product.
- Description: This data attribute contains the description about the item.
- Quantity: This data attribute contains the quantities for each product per transaction. The data is in a numeric format.
- InvoiceDate: The data attribute contains the invoice date and time. It indicates the day and time when each transaction was generated.
- UnitPrice: The price indicates the product price per unit in sterling.
- CustomerID: This column has the customer identification number. It is a five-digit integer number uniquely assigned to each customer.
- Country: This column contains the geographic information about the customer. It records the country name for the customers.
You can refer to the sample of the dataset given in the following screenshot:

Figure 3.4: Sample recodes from the dataset
Now we will start building the customer segmentation application.