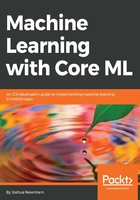
A brief introduction to Core ML
With the release of iOS 11 and Core ML, performing inference is just a matter of a few lines of code.Prior to iOS 11, inference was possible, but it required some work to take a pre-trained model and port it across using an existing framework such as Accelerate or metal performance shaders (MPSes).Accelerate and MPSes are still used under the hood by Core ML, but Core ML takes care of deciding which underlying framework your model should use (Accelerate using the CPU for memory-heavy tasks and MPSes using the GPU for compute-heavy tasks). It also takes care of abstracting a lot of the details away; this layer of abstraction is shown in the following diagram:

There are additional layers too; iOS 11 has introduced and extended domain-specific layers that further abstract a lot of the common tasks you may use when working with image and text data, such as face detection, object tracking, language translation, and named entity recognition (NER). These domain-specific layers are encapsulated in theVision and natural language processing (NLP) frameworks; we won't be going into any details of these frameworks here, but you will get a chance to use them in later chapters:

It's worth noting that these layers are not mutually exclusive and it is common to find yourself using them together, especially the domain-specific frameworks that provide useful preprocessing methods we can use to prepare our data before sending to a Core ML model.
So what exactly is Core ML? You can think of Core ML as a suite of tools used to facilitate the process of bringing ML models to iOS and wrapping them in a standard interface so that you can easily access and make use of them in your code. Let's now take a closer look at the typical workflow when working with Core ML.