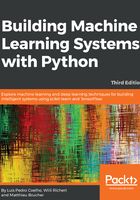
Summary
In this chapter, we started with the oldest trick in the book: ordinary least squares regression. Although centuries old, it is sometimes still the best solution for regression. However, we also saw more modern approaches that avoid overfitting and can give us better results, especially when we have a large number of features. We used Ridge, Lasso, and ElasticNets; these are state-of-the-art methods for regression.
We saw, once again, the danger of relying on training errors to estimate generalization: it can be an overly optimistic estimate to the point where our model has zero training errors, but we know that it is completely useless. When thinking through these issues, we were led on to two-level cross-validation, an important area that many in the field still have not completely internalized.
Throughout this chapter, we were able to rely on scikit-learn to support all the operations we wanted to perform, including an easy way to achieve correct cross-validation. ElasticNets with an inner cross-validation loop for parameter optimization (as implemented in scikit-learn by ElasticNetCV) should probably become your default method for regression. We also saw the usage of TensorFlow for regression (so the package is not restricted to neural network computations).
One reason to use an alternative is when you are interested in a sparse solution. In this case, a pure Lasso solution is more appropriate as it will set many coefficients to zero. It will also allow you to discover, from the data, a small number of variables, which are important to the output. Knowing the identity of these may be interesting in and of itself, in addition to having a good regression model.
Chapter 4, Classification I – Detecting Poor Answers, looks at how to proceed when your data does not have predefined classes for classification.