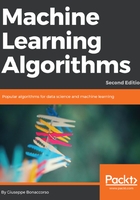
Feature selection and filtering
An unnormalized dataset with many features contains information proportional to the independence of all features and their variance. Let's consider a small dataset with three features, generated with random Gaussian distributions:

Even without further analysis, it's obvious that the central line (with the lowest variance) is almost constant and doesn't provide any useful information. Recall from Chapter 2, Important Elements in Machine Learning, that the entropy H(X) is quite small, while the other two variables carry more information. A variance threshold is, therefore, a useful approach to remove all those elements whose contribution (in terms of variability and so, information) is under a predefined level. The scikit-learn library provides the VarianceThreshold class which can easily solve this problem. By applying it to the previous dataset, we get the following result:
from sklearn.feature_selection import VarianceThreshold
X[0:3, :]
array([[-3.5077778 , -3.45267063, 0.9681903 ],
[-3.82581314, 5.77984656, 1.78926338],
[-2.62090281, -4.90597966, 0.27943565]])
vt = VarianceThreshold(threshold=1.5)
X_t = vt.fit_transform(X)
X_t[0:3, :]
array([[-0.53478521, -2.69189452],
[-5.33054034, -1.91730367],
[-1.17004376, 6.32836981]])
The third feature has been completely removed because its variance is under the selected threshold (1.5, in this case).
There are also many univariate methods that can be used in order to select the best features according to specific criteria based on F-tests and p-values, such as chi-square or Analysis of Variance (ANOVA). However, their discussion is beyond the scope of this book and the reader can find further information in Statistics for Machine Learning, Dangeti P., Packt Publishing, 2017.
Two examples of feature selection that use the SelectKBest class (which selects the best K high-score features) and the SelectPercentile class (which selects only a subset of features belonging to a certain percentile) are shown next. It's possible to apply them both to regression and classification datasets, being careful to select appropriate score functions:
from sklearn.datasets import load_boston, load_iris
from sklearn.feature_selection import SelectKBest, SelectPercentile, chi2, f_regression
regr_data = load_boston()
print(regr_data.data.shape)
(506L, 13L)
kb_regr = SelectKBest(f_regression)
X_b = kb_regr.fit_transform(regr_data.data, regr_data.target)
print(X_b.shape)
(506L, 10L)
print(kb_regr.scores_)
array([ 88.15124178, 75.2576423 , 153.95488314, 15.97151242,
112.59148028, 471.84673988, 83.47745922, 33.57957033,
85.91427767, 141.76135658, 175.10554288, 63.05422911,
601.61787111])
class_data = load_iris()
print(class_data.data.shape)
(150L, 4L)
perc_class = SelectPercentile(chi2, percentile=15)
X_p = perc_class.fit_transform(class_data.data, class_data.target)
print(X_p.shape)
(150L, 1L)
print(perc_class.scores_)
array([ 10.81782088, 3.59449902, 116.16984746, 67.24482759])