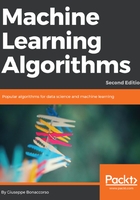
Class balancing
When working with the majority of machine learning algorithms (in particular, supervised ones), it's important to train the model with a dataset containing almost the same number of elements for each class. Remember that our goal is training models that can generalize in the best way for all of the possible classes and supposes that we have a binary dataset containing 1,000 samples with a proportion (0.95, 0.05). There are many scenarios where this proportion is very common. For example, a spam detector can collect lots of spam emails, but it's much more difficult to have access to personally accepted emails. However, we can suppose that some users (a very small percentage) decided to share anonymous regular messages so that our dataset consists of 5% non-spam entries.
Now, let's consider a static algorithm that always outputs the label 0 (for example, due to a bug). Which is the final accuracy? In a similar situation, we would expect a behavior similar to a random oracle based on a coin flip, but, not surprisingly, we discover that this algorithm reaches an accuracy of 95%! How is this possible? Simply because the dataset is unbalanced and the number of samples with label 1 is negligible with respect to the total dataset size. For this reason, before any training process, it's important to perform a basic descriptive analysis of the data, trying to correct the balancing problems.