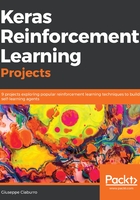
SARSA
The State-action-reward-state-action (SARSA) algorithm implements an on-policy time differences method, in which the update of the action-value function is performed based on the outcome of the transition from state s to state s' through action a, based on a selected policy π (s, a).
There are policies that always choose the action that provides the maximum reward and non-deterministic policies (ε-greedy, ε-soft, softmax), which ensure an element of exploration in the learning phase.
In SARSA, it is necessary to estimate the action-value function q (s, a), because the total value of a state v (s) (value function) is not sufficient in the absence of an environment model to allow the policy to determine, given a state, which action is best performed. In this case, however, the values are estimated step by step following the Bellman equation with the update parameter v (s), considering, however, in place of a state, the state-action pair.
Being of an on-policy nature, SARSA estimates the action-value function based on the behavior of the π policy, and at the same time modifies the greedy behavior of the policy with respect to the updated estimates from the action-value function. The convergence of SARSA, and more generally of all TD methods, depends on the nature of policies.
The following is a pseudocode for the SARSA algorithm:
Initialize
arbitrary action-value function
Repeat (for each episode)
Initialize s
choose a from s using policy from action-value function
Repeat (for each step in episode)
take action a
observe r, s'
choose a' from s' using policy from action-value function
update action-value function
update s,a
The update rule of the action-value function uses all five elements (st, at, rt + 1, st + 1, at + 1); for this reason, it is called SARSA.