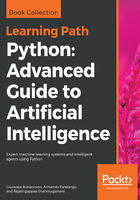
Metropolis-Hastings sampling
We have seen that the full joint probability distribution of a Bayesian network P(x1, x2, x3, ..., xN) can become intractable when the number of variables is large. The problem can become even harder when it's needed to marginalize it in order to obtain, for example, P(xi), because it's necessary to integrate a very complex function. The same problem happens when applying the Bayes' theorem in simple cases. Let's suppose we have the expression p(A|B) = K · P(B|A)P(A). I've expressly inserted the normalizing constant K, because if we know it, we can immediately obtain the posterior probability; however, finding it normally requires integrating P(B|A)P(A), and this operation can be impossible in closed form.
The Metropolis-Hastings algorithm can help us in solving this problem. Let's imagine that we need to sample from P(x1, x2, x3, ..., xN), but we know this distribution up to a normalizing constant, so P(x1, x2, x3, ..., xN) ∝ g(x1, x2, x3, ..., xN). For simplicity, from now on we collapse all variables into a single vector, so P(x) ∝ g(x).
Let's take another distribution q(x'|x(i-1)), which is called candidate-generating distribution. There are no particular restrictions on this choice, only that q is easy to sample. In some situations, q can be chosen as a function very similar to the distribution p(x), which is our target, while in other cases, it's possible to use a normal distribution with mean equal to x(i-1). As we're going to see, this function acts as a proposal-generator, but we're not obliged to accept all the samples drawn from it therefore, potentially any distribution with the same domain of P(X) can be employed. When a sample is accepted, the Markov chain transitions to the next state, otherwise it remains in the current one. This decisional process is based on the idea that the sampler must explore the most important state-space regions and discard the ones where the probability to find good samples is low.
The algorithm proceeds with the following steps:
- Initialize the variable NIterations
- Initialize x(0) randomly
- For t=1 to NIterations:
- Draw a candidate sample x' from q(x'|x(i-1))
- Compute the following value:
- If α ≥ 1:
- Accept the sample x(t) = x'
- Else if 0 < α < 1:
- Accept the sample x(t) = x' with probability α; or
- Reject the sample x' setting x(t) = x(t-1) with probability 1 - α
It's possible to prove (the proof will be omitted, but it's available in Markov Chain Monte Carlo and Gibbs Sampling, Walsh B., Lecture Notes for EEB 596z) that the transition probability of the Metropolis-Hastings algorithm satisfies the detailed balance equation, and therefore the algorithm converges to the true posterior distribution.