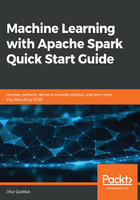
Datasets
Datasets extend DataFrames by providing type safety. This means that in the preceding example of the missing column, the Dataset API will throw a compile time error. In fact, DataFrames are actually an alias for Dataset[Row] where a Row is an untyped object that you may see in Spark applications written in Java. However, because R and Python have no compile-time type safety, this means that Datasets are not currently available to these languages.
There are numerous advantages to using the DataFrame and Dataset APIs over RDDs, including better performance and more efficient memory usage. The high-level APIs offered by DataFrame and Dataset also make it easier to perform standard operations such as filtering, grouping, and calculating statistical aggregations such as totals and averages. RDDs, however, are still useful because of the greater degree of control offered by its low-level API, including low-level transformations and actions. They also provide analysis errors at compile time and are well suited to unstructured data.