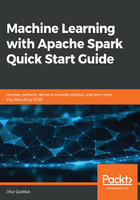
Distributed data stores
Let's return to the problems faced by a single-machine RDBMS. We have seen how sharding can be employed as one method to scale relational databases horizontally in order to optimize costs as data grows for small- to medium-sized databases. However, the issue with sharding is that each node acts in a standalone manner with no knowledge of the other nodes in the cluster, meaning that a custom broker is required to both partition the data across the shards and to process read and write requests.
Distributed data stores, on the other hand, work out of the box as a single logical unit spanning a cluster of nodes.
Client applications view the distributed data store as a single entity, meaning that no matter which node in the cluster physically handles the client request, the same results will be returned. Distributed filesystems, such as the Apache Hadoop Distributed File System (HDFS) discussed in the next section, belong to the class of distributed data stores and are used to store files in their raw format. When data needs to be modeled in some manner, then distributed databases can be used. Depending on the type of distributed database, it can either be deployed on top of a distributed filesystem or not.