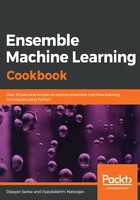
How it works...
In Step 1, we imported the libraries that are required to build our models. In Step 2, we created the response and feature sets. We retrieved our feature set using the iloc() function of the pandas DataFrame. In Step 3, we split our dataset into training and testing sets. In Step 4, we built our base classifiers. Kindly note that we passed probability=True to our SVC function to allow SVC() to return class probabilities. In the SVC class, the default is probability=False.
In Step 5, we fitted our model to the training data. We used the predict_proba() function in Step 6 to predict the class probabilities for our test observations.
Finally, in Step 7, we assigned different weights to each of our models to estimate the weighted average predictions. The question that comes up is how to choose the weights. One way is to sample the weights uniformly and to make sure they normalize to one and validate on the test set and repeat keeping track of weights that provide the highest accuracy. This is an example of a random search.