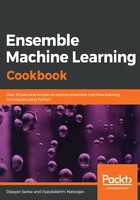
上QQ阅读APP看书,第一时间看更新
How to do it...
We have a dataset that is based on the properties of wines. Using this dataset, we'll build multiple regression models with the quality as our response variable. With multiple learners, we extract multiple predictions. The averaging technique would take the average of all of the predicted values for each training sample:
- Import the required libraries:
# Import required libraries
from sklearn.linear_model import LinearRegression
from sklearn.tree import DecisionTreeRegressor
from sklearn.svm import SVR
- Create the response and feature sets:
# Create feature and response variable set
from sklearn.cross_validation import train_test_split
# create feature & response variables
feature_columns = ['fixed acidity', 'volatile acidity', 'citric acid', 'residual sugar','chlorides', 'free sulfur dioxide', 'total sulfur dioxide','density', 'pH', 'sulphates', 'alcohol']
X = df_winedata[feature_columns]
Y = df_winedata['quality']
- Split the data into training and testing sets:
# Create train & test sets
X_train, X_test, Y_train, Y_test = \
train_test_split(X, Y, test_size=0.20, random_state=1)
- Build the base regression learners using linear regression, SVR, and a decision tree:
# Build base learners
linreg_model = LinearRegression()
svr_model = SVR()
regressiontree_model = DecisionTreeRegressor()
# Fitting the model
linreg_model.fit(X_train, Y_train)
svr_model.fit(X_train, Y_train)
regressiontree_model.fit(X_train, Y_train)
- Use the base learners to make a prediction based on the test data:
linreg_predictions = linreg_model.predict(X_test)
svr_predictions = svr_model.predict(X_test)
regtree_predictions = regressiontree_model.predict(X_test)
- Add the predictions and divide by the number of base learners:
# We divide the summation of the predictions by 3 i.e. number of base learners
average_predictions=(linreg_predictions + svr_predictions + regtree_predictions)/3