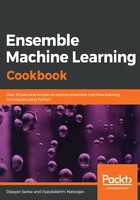
上QQ阅读APP看书,第一时间看更新
There's more...
Many classifiers can estimate class probabilities. In this case, the class labels are predicted by averaging the class probabilities. This is called soft voting and is recommended for an ensemble of well-tuned classifiers.
In the scikit-learn library, many classification algorithms have the predict_proba() method to predict the class probabilities. To perform the ensemble with soft voting, simply replace voting='hard' with voting='soft' in VotingClassifier().
The following code creates an ensemble using soft voting:
# create the sub models
estimators = []
dt_model = DecisionTreeClassifier(random_state=1)
estimators.append(('DecisionTree', dt_model))
svm_model = SVC(random_state=1, probability=True)
estimators.append(('SupportVector', svm_model))
logit_model = LogisticRegression(random_state=1)
estimators.append(('Logistic Regression', logit_model))
for each_estimator in (dt_model, svm_model, logit_model):
each_estimator.fit(X_train, Y_train)
Y_pred = each_estimator.predict(X_test)
print(each_estimator.__class__.__name__, accuracy_score(Y_test, Y_pred))
# Using VotingClassifier() to build ensemble model with Soft Voting
ensemble_model = VotingClassifier(estimators=estimators, voting='soft')
ensemble_model.fit(X_train,Y_train)
predicted_labels = ensemble_model.predict(X_test)
print("Classifier Accuracy using Soft Voting: ", accuracy_score(Y_test, predicted_labels))
We get to see the accuracy from individual learners and the ensemble learner using soft voting:

The SVC class can't estimate class probabilities by default, so we've set its probability hyper-parameter to True in the preceding code. With probability=True, SVC will be able to estimate class probabilities.