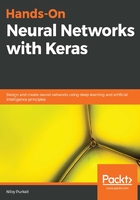
A single layered network
Right, now we have seen how to leverage two versions of our perception unit, in parallel, enabling each individual unit to learn a different underlying pattern that is possibly present in the data we feed it. We naturally want to connect these neurons to output neurons, which fire to indicate the presence of a specific output class. In our sunny-rainy day classification example, we have two output classes (sunny or rainy), hence a predictive network tasked to solve this problem will have two output neurons. These neurons will be supported by the learning of neurons from the previous layer, and ideally will represent features that are informative for predicting either a rainy or a sunny day. Mathematically speaking, all that is simply happening here is the forward propagation of our transformed input features, followed by the backward propagation of the errors in our prediction. One way of thinking about this is to visualize each node in the following diagram as the holder of a specific number. Similarly, each arrow can be seen as picking up a number from a node, performing a weighted computation on it, and carrying it forward to the next layer of nodes:

Now, we have a neural network with one hidden layer. We call this a hidden layer as the state of this layer is not directly enforced, as opposed to the input and output layers. Their representations are not hardcoded by the designer of the network. Rather, they are inferred by the network, as data propagates through it.
As we saw, the input layer holds our input values. The set of arrows connecting the input layer to the hidden layer simply compute the bias adjusted dot product (z), of our input features (x) and their respective weights (θ1). The (z) values then reside in the hidden layer neurons, until we apply our non-linear function, g(x), to these values. After this, the arrows leading away from the hidden layer compute the dot product of g(z) and the weights corresponding to the hidden layer, (θ2), before carrying the result forward to the two output neurons, and
. Notice that with each layer comes a respective weights matrix, which is iteratively updated by differentiating our loss function with respect to the weight matrices from the previous training iteration. Hence, we train a neural network by descending the gradient of our loss function relative to our model weights, converging to a global minimum.