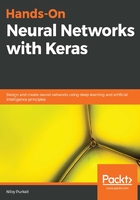
Underfitting
You have collected about two months worth of air pressure data, and balanced the number of observations in each of your output classes. Your prediction accuracy has steadily increased, but starts tapering off at a suboptimal level (let's say 61%). Suddenly, your model's accuracy starts dropping again, as it gets colder and colder outside. Here, we face the problem of underfitting, as our simplistic model is unable to capture the underlying pattern of our data, caused by the seasonal coming of winter. There are a few simple remedies to this situation. Most prominently, we may simply improve our model by adding more predictive features, such as the outside temperature. Doing so, we observe after a few days of data collection that once again, our accuracy climbs up, as the additional feature adds more information to our model, increasing its predictive power. In other cases of underfitting, we may well have chosen to select a more computationally-intensive predictive model, add more data and engineer better features, or reduce any mathematical constraints (such as the lambda hyperparameter for regularization) on the model.