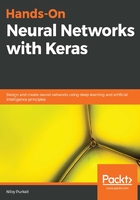
Representing information
Consider a task where you have to correctly classify images of dogs, cats, and attack helicopters. One way of thinking about a neuronal learning system is that we dedicate several neurons to represent the various features that exist in the three respective classes. In other words, let's say that we have employed three expert neurons for our classification task here. Each one of these neurons is an expert in the domain of what a dog, cat, and an attack helicopter looks like.
How are they experts? Well, for now, we can think that each of our domain expert neurons are supported by their own cabinet of employees and support staff, all diligently working for these experts, collecting and representing different breeds of dogs, cats, and attack helicopters, respectively. But we don't deal with their support staff for the time being. At the moment, we simply present any image to each of our three domain experts. If the picture is of a dog, our dog expert neuron immediately recognizes the creature and fires, almost as if it were saying, Hello, I believe this is a dog. Trust me, I'm an expert. Similarly, when we present our three experts a picture of a cat, our cat neuron will signal to us that they have detected a cat in our image by firing. While this is not exactly how each neuron represents real-world objects, such as cats and dogs, it helps us gain a functional understanding of neuron-based learning systems. Hopefully, you have enough information now to be introduced to the biological neuron's less sophisticated brother, the artificial neuron, next.