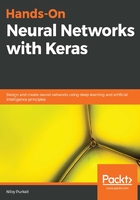
Language processing
So far, we have seen how we can train a simple feedforward neural network on Keras for an image classification task. We also saw how we can mathematically represent image data as a high-dimensional geometric shape, namely a tensor. We saw that a higher-order tensor is simply composed of tensors of a smaller order. Pixels group up to represent an image, which in turn group up to represent an entire dataset. Essentially, whenever we want to employ the learning mechanism of neural networks, we have a way to represent our training data as a tensor. But what about language? How can we represent human thought, with all of its intricacies, as we do through language? You guessed it—we will use numbers once again. We will simply translate our texts, which are composed of sentences, which themselves are composed of words, into the universal language of mathematics. This is done through a process known as vectorization, which we will explore first-hand during our task of classifying the sentiment of movie reviews by using the internet movie database (IMDB) dataset.