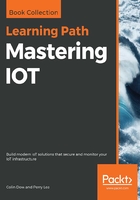
Sensor fusion
One aspect that needs consideration with all the sensor devices described in this chapter is the concept of sensor fusion. Sensor fusion is the process of combining several different kinds of sensor data to reveal more about context than a single sensor can provide. This is important in the IoT space, since a single thermal sensor has no notion of what causes a rapid temperature change. However, when combined with data from other sensors nearby that look at PIR motion detection and light intensity, an IoT system could discern that a large number of people are congregating in a certain area while the sun is shining, and could then make the decision to increase air circulation in a smart building. A simple thermal sensor only records the current temperature value, and has no contextual awareness that the heat is rising due to people congregating and sunlight shining.
With time-correlated data from multiple sensors (edge and cloud), processing can make better decisions based on more data. This is one of the reasons that there will be a large influx of data from sensors to the cloud, and this is causing big data growth. As sensors become cheaper and easier to integrate, as with the TI SensorTag, we will see more combined sensing to provide contextual awareness.
There are two modes of sensor fusion:
- Centralized: Where raw data is streamed and aggregated to a central service and fusion occurs there (cloud-based, for example)
- De-centralized: Where data is correlated at the sensor (or close to it)
The basis of correlating sensor data is usually expressed through the central limit theorem, where two sensor measurements, x1 and x2, are combined to reveal a correlated measurement, x3, based on the combined variances. This is simply adding two measures, and weighting the sum by the variances:

Other sensor fusion methods used are Kalman filters and Bayesian networks.