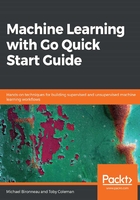
What is ML?
ML is a field at the intersection of statistics and computer science. The output of this field has been a collection of algorithms capable of operating autonomously by inferring the best decision or answer to a question from a dataset. Unlike traditional programming, where the programmer must decide the rules of the program and painstakingly encode these in the syntax of their chosen programming language, ML algorithms require only sufficient quantities of prepared data, computing power to learn from the data, and often some knowledge to tweak the algorithms parameters to improve the final result.
The resulting systems are very flexible and can be excellent at capitalizing on patterns that human beings would miss. Imagine writing a recommender system for a TV series from scratch. Perhaps you might begin by defining the inputs and the outputs of the problem, then finding a database of TV series that had such details as their date of release, genre, cast, and director. Finally, you might create a score func that rates a pair of series more highly if their release dates are close, they have the same genre, share actors, or have the same director.
Given one TV series, you could then rank all other TV series by decreasing similarity score and present the first few to the user. When creating the score func, you would make judgement calls on the relative importance of the various features, such as deciding that each pair of shared actors between two series is worth one point. This type of guesswork, also known as a heuristic, is what ML algorithms aim to do for you, saving time and improving the accuracy of the final result, especially if user preferences shift and you have to change the scoring func regularly to keep up.
The distinction between the broader field of AI and ML is a murky one. While the hype surrounding ML may be relatively new[6], the history of the field began in 1959 when Arthur Samuel, a leading expert in AI, first used these words[7]. In the 1950s, ML concepts such as the perceptron and genetic algorithms were invented by the likes of Alan Turing[8] as well as Samuel himself. In the following decades, practical and theoretical difficulties in achieving general AI, led to approaches such as rule-based methods such as expert systems, which did not learn from data, but rather from expert-devised rules which they had learned over many years, encoded in if-else statements.
In the 1990s, recognizing that achieving AI was unlikely with existing technology, there was an increasing appetite for a narrow approach to tackling very specific problems that could be solved using a combination of statistics and probability theory. This led to the development of ML as a separate field. Today, ML and AI are often used interchangeably, particularly in marketing literature[9].