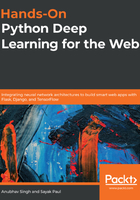
Spam filtering
When half the emails being sent across the world are marked spam, it's an issue. While at first thought, we associate fraudulent and unnecessary emails promoting businesses and products as spam, that's only a part of the definition. It is important to realize that even good, quality content when posted on the same document several times over is spam. Furthermore, the web has evolved since the term spam was first used in Usenet groups. What was initially an activity performed with the intention of annoying people, or driving in messages forcefully to certain target users, spam today is much more evolved and potentially a lot more dangerous—from being able to track your browser activity to identity theft, there is a lot of malicious spam on the internet today that compromises user security and privacy.
Today, we have spam of various kinds—instant messenger spam, website spam, advertisement spam, SMS spam, social media spam, and many other forms.
Apart from a few, most types of spam are exhibited on the internet. It is hence critical to be able to filter spam and take protective measures against it. While the most initial spam-fighting began as early as the 1990s with identifying the IP addresses that were sending out spam emails, it was soon realized to be a highly inefficient method to do so as the blacklist grew large and its distribution and maintenance became a pain.
In the early 2000s, when Paul Graham published a paper titled A Plan for Spam, for the first time, an ML model—Bayesian filtering—was deployed to fight spam. Soon, several spam-fighting tools were spun from the paper and proved to be efficient.
Such was the impact of Bayesian filtering method against spam that, at the World Economic Forum in 2004, the founder of Microsoft, Bill Gates went forward to say that:
Bill Gates, however, as we know today, could not have been more wrong in this one prediction. Spam evolved, with spammers studying Bayesian filtering and finding out ways to avoid being marked as spam in the detection phase. Today, neural networks are deployed on large scale, continuously scanning new emails and taking decisions on determining spam or non-spam content, which could not have been logically reached by a human by merely studying logs of email spam.