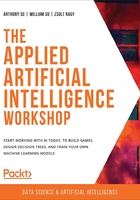
Introduction
In the previous chapter, you were introduced to the fundamentals of Artificial Intelligence (AI), which helped you create the game Tic-Tac-Toe. In this chapter, we will be looking at regression, which is a machine learning algorithm that can be used to measure how closely related independent variable(s), called features, relate to a dependent variable called a label.
Linear regression is a concept with many applications a variety of fields, ranging from finance (predicting the price of an asset) to business (predicting the sales of a product) and even the economy (predicting economy growth).
Most of this chapter will deal with different forms of linear regression, including linear regression with one variable, linear regression with multiple variables, polynomial regression with one variable, and polynomial regression with multiple variables. Python provides lots of forms of support for performing regression operations and we will also be looking at these later on in this chapter.
We will also use an alternative regression model, called Support Vector Regression (SVR), with different forms of linear regression. Throughout this chapter, we will be using a few sample datasets along with the stock price data loaded from the Quandl Python library to predict future prices using different types of regression.
Note
Although it is not recommended that you use the models in this chapter to provide trading or investment advice, this is a very exciting and interesting journey that explains the fundamentals of regression.