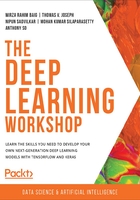
Introduction
In the previous chapters, we learned about traditional neural networks and a number of models, such as the perceptron. We learned how to train such models on structured data for regression or classification purposes. Now, we will learn how we can extend their application to the computer vision field.
Not so long ago, computers were perceived as computing engines that could only process well-defined and logical tasks. Humans, on the other hand, are more complex since we have five basic senses that help us see things, hear noises, feel things, taste foods, and smell odors. Computers were only calculators that could operate large volumes of logical operations, but they couldn't deal with complex data. Compared to the abilities of humans, computers had very clear limitations.
There were some rudimentary attempts to “give sight" to computers by processing and analyzing digital images. This field is called computer vision. But it was not until the advent of deep learning that we saw some incredible improvements and results. Nowadays, the field of computer vision has advanced to such an extent that, in some cases, computer vision AI systems are able to process and interpret certain types of images faster and more accurately than humans. You may have heard about the experiment where a group of 15 doctors in China competed against a deep learning system from the company BioMind AI for recognizing brain tumors from X-rays. The AI system took 15 minutes to accurately predict 87% of the 225 input images, while it took 30 minutes for the medical experts to achieve a score of 66% on the same pool of images.
We've all heard about self-driving cars that can automatically make the right decisions depending on traffic conditions or drones that can detect sharks and automatically send alerts to lifeguards. All these amazing applications are possible thanks to the recent development of CNNs.
Computer vision can be split into four different domains:
- Image classification, where we need to recognize the main object in an image.
- Image classification and localization, where we need to recognize and localize the main object in an image with a bounding box.
- Object detection, where we need to recognize multiple objects in an image with bounding boxes.
- Image segmentation, where we need to identify the boundaries of objects in an image.
The following figure shows the difference between the four domains:

Figure 3.1: Difference between the four domains of computer vision
In this chapter, we will only look at image classification, which is the most widely used application of CNN. This includes things such as car plate recognition, automatic categorization of the pictures taken with your mobile phone, or creating metadata used by search engines on databases of images.
Note
If you're reading the print version of this book, you can download and browse the color versions of some of the images in this chapter by visiting the following link: https://packt.live/2ZUu5G2