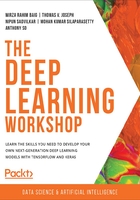
Activity 2.01: Build a Multilayer Neural Network to Classify Sonar Signals
In this activity, we will use the Sonar dataset (https://archive.ics.uci.edu/ml/datasets/Connectionist+Bench+(Sonar,+Mines+vs.+Rocks)), which has patterns obtained by bouncing sonar signals off a metal cylinder at various angles and under various conditions. You will build a neural network-based classifier to classify between sonar signals bounced off a metal cylinder (the Mine class), and those bounced off a roughly cylindrical rock (the Rock class). We recommend using the Keras API to make your code more readable and modular, which will allow you to experiment with different parameters easily:
Note
You can download the sonar dataset from this link https://packt.live/31Xtm9M.
- The first step is to understand the data so that you can figure out whether this is a binary classification problem or a multiclass classification problem.
- Once you understand the data and the type of classification that needs to be done, the next step is network configuration: the number of neurons, the number of hidden layers, which activation function to use, and so on.
Recall the network configuration steps that we've covered so far. Let's just reiterate a crucial point, the activation function part: for the output (the last) layer, we use sigmoid to do binary classification and Softmax to do multiclass classification.
- Open the sonar.csv file to explore the dataset and see what the target variables are.
- Separate the input features and the target variables.
- Preprocess the data to make it neural network-compatible. Hint: one-hot encoding.
- Define a neural network using Keras and compile it with the right loss function.
- Print out a model summary to verify the network parameters and considerations.
You are expected to get an accuracy value above 95% by designing a proper multilayer neural network using these steps.
Note
The detailed steps for this activity, along with the solutions and additional commentary, are presented on page 390.